Urban and rural scenarios issue major challenges to partially, highly and fully automated vehicles. In particular, the multitude of different, complex environments, road users and environmental conditions requires accurate and reliable environment sensing by the in-vehicle environment sensors camera, LiDAR and radar. In addition to being used for driver assistance systems and automated driving systems, predictive environment awareness can also be used to further improve the robustness of passive and integral safety systems and extend their functionality. If a collision is unavoidable, reversible and irreversible safety systems are triggered early to reduce or avoid injuries or fatalities to all road users. Comprehensive requirements for environment perception in the near field such as minimal error rate and high accuracy are mandatory for this. Consequently, it is essential to realize a fast, reliable and trustworthy environment detection in all traffic situations. Challenges include accurate and detailed object detection, minimizing cycle times, and safeguarding environment detection when individual sensors are disturbed by rain, for example.
Environmental sensors
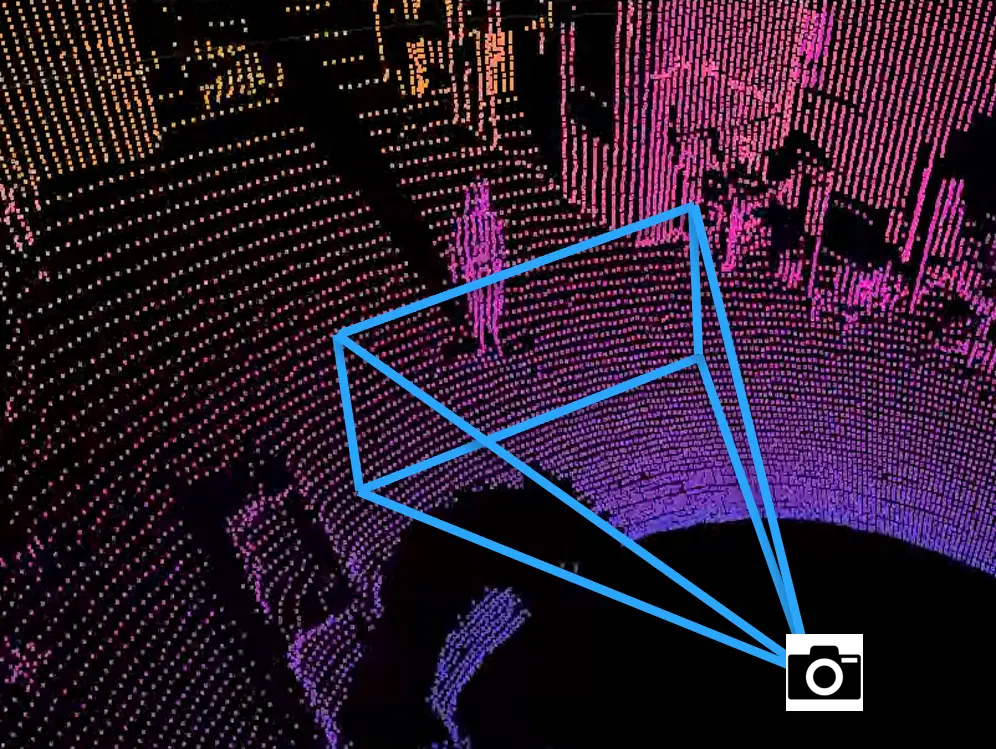
With the help of radar, camera and LiDAR, modern vehicles can perceive the vehicle's surroundings. All three sensor technologies work with different measurement principles, which means they have different strengths and weaknesses. Radar, for example, is robust in the face of weather conditions, but object detection can be disturbed by multiple reflections and the resulting ghost objects. Among other things, the camera is very good for object classification, but it is susceptible to overexposure and underexposure, such as when the sun is low in the sky. LiDAR, for example, allows accurate distance measurement, but is disturbed by weather. C-ISAFE takes advantage of the specific characteristics and researches methods to improve the performance of individual sensors as well as sensor systems. The focus here is on robust and detailed detection in the near field for vehicle safety systems.
Detailed object detection in the pre-crash phase

The steadily increasing resolution and performance of environmental sensors enables a progressive improvement in object detection quality and detail. Especially in the pre-crash phase, when the distance between sensors and potential collision objects is small, additional information can be collected and evaluated. For example, in the event of a (potential) crash with another vehicle, accurate determination of relevant parameters such as size, distance, angle of approach and speed is required to enable reliable prediction of the accident event. Of particular interest are object features whose position is statically located within the object, e.g., the position of the vehicle wheels. These salient points enable reliable and accurate object detection and tracking. In C-ISAFE, a radar-based method has been developed and patented to determine and track the position and speed of rotating vehicle wheels based on the micro-Doppler effect. This approach of determining prominent parts of a vehicle was adapted and extended by a feature-based extraction methodology using an AI method for camera sensor technology. Combinatorially, crash-relevant object parameters of the entire vehicle can be determined from this. By means of LiDAR, supplementary information about the geometry of objects can be determined, such as a contour determination of vehicles. In addition to the detection of vehicles with regard to verhicle-vehicle crashes, other road users will be considered object-specifically. The research team is also focusing on pedestrian protection.
Robust object tracking
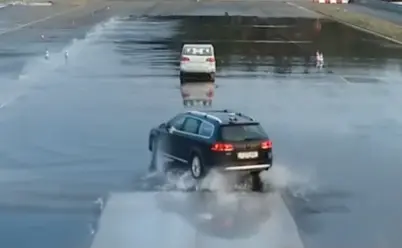
In order to be able to activate future safety systems by using predictive sensors, the near vehicle environment must be reliably and continuously detected. In addition, the activation of irreversible, passive safety systems represents a safety-critical decision and is classified in the highest protection level. Therefore, in addition to the development of sensor-specific methods, one focus of the research team is to improve object detection, especially in terms of robustness, through sensor fusion. AI algorithms can be used to fuse sensors at different levels. In particular, a deep-learning architecture for early fusion is being developed in C-ISAFE in order to be able to optimally take into account the different effects of disturbance factors on the individual sensors. The current research topic is safety under adverse weather conditions. In addition, safeguarding concepts and independent plausibility methods of predictive sensors are being developed to increase robustness and safety.
Almost every sixth accident of a passenger car with injured occupants shows skidding in the pre-crash phase despite modern ESP systems. Causes include uncoordinated driver action in safety-critical driving situations or a slippery road surface caused by snow and ice. Skidding represents a special, nonlinear vehicle motion that strongly depends on external environmental conditions, such as the friction coefficient of the road surface.
In C-ISAFE, new methods for object detection and tracking in critical driving situations such as skidding are being researched, implemented in prototype vehicles and tested under real environmental conditions.
Publications
- R. Huber, K. Schneider, A. Wetzel, E. Neitzel, and T. Brandmeier, "Light Analysis for Optimized Object Detection with Cameras for Integrated Safety Systems," in 2022 International Conference on Electrical, Computer and Energy Technologies (ICECET), Prague, Czech Republic, 2022, pp. 1–6, doi: 10.1109/ICECET55527.2022.9872761.
- D. M. Mothershed, R. Lugner, S. Afraj, G. J. Sequeira, K. Schneider, T. Brandmeier, and V. Soloiu, "Comparison and Evaluation of Algorithms for LiDAR-Based Contour Estimation in Integrated Vehicle Safety," IEEE Trans. Intell. Transport. Syst., vol. 23, no. 5, pp. 3925–3942, 2022, doi: 10.1109/TITS.2020.3044753.
- A. Kamann, D. Steinhauser, F. Gruson, T. Brandmeier, and U. Schwarz, "Extended Object Tracking Using Spatially Resolved Micro-Doppler Signatures," IEEE Transactions on Intelligent Vehicles, vol. 6, no. 3, pp. 440–449, 2020, doi: 10.1109/TIV.2020.3035433.
- D. Vriesman, M. E. Pederiva, J. Mario De Martino, A. B. Junior, A. Zimmer, and T. Brandmeier, "A Fusion Approach for Pre-Crash Scenarios based on Lidar and Camera Sensors," in 2021 IEEE 93rd Vehicular Technology Conference (VTC2021-Spring), Helsinki, Finland, 2021, pp. 1–5, doi: 10.1109/VTC2021-Spring51267.2021.9449039.
- G. J. Sequeira, B. Harlapur, D. O. Ortegon, R. Lugner, T. Brandmeier, and V. Soloiu, "Investigation of Influence from Variation in Color on LiDAR Sensor for Perception of Environment in Autonomous Vehicles," in 2021 International Symposium ELMAR, 2021, Zadar, Croatia, pp. 71–76, doi: 10.1109/ELMAR52657.2021.9550943.
- P. Held, D. Steinhauser, A. Koch, T. Brandmeier, and U. T. Schwarz, "A Novel Approach for Model Based Pedestrian Tracking Using Automotive Radar," IEEE Trans. Intell. Transport. Syst., vol. 23, no. 7, pp. 7082–7095, 2022, doi: 10.1109/TITS.2021.3066680.
- G. J. Sequeira, M. Surve, S. Afraj, and T. Brandmeier, “A novel concept for validation of pre-crash perception sensor information using contact sensor,” in 2020 IEEE 23rd International Conference on Intelligent Transportation Systems (ITSC), Rhodes, Greece, 2020, pp. 1-7, doi: 10.1109/ITSC45102.2020.9294242.
- G. J. Sequeira, S. Afraj, M. Surve, and T. Brandmeier, “LiDAR point cloud analysis for vehicle contour estimation using polynomial approximation and curvature breakdown,” in 2020 IEEE 92nd Vehicular Technology Conference (VTC2020-Fall), Victoria, BC, Canada, 2020, pp. 1-6, doi: 10.1109/VTC2020-Fall49728.2020.9348457.
- R. Lugner, D. Vriesman, M. Inderst, G. J. Sequeira, N. Pasupuleti, A. Zimmer, and T. Brandmeier, “Evaluation of Sensor Tolerances and Inevitability for Pre-Crash Safety Systems in Real Case Scenarios,” in 2020 IEEE 3rd Connected and Automated Vehicles Symposium (CAVS), Victoria, BC, Canada, 2020, pp. 1-6, doi: 10.1109/CAVS51000.2020.9334578.
- D. Vriesman, B. Thoresz, D. Steinhauser, A. Zimmer, A. Britto, and T. Brandmeier, "An Experimental Analysis of Rain Interference on Detection and Ranging Sensors," in 2020 IEEE 23rd International Conference on Intelligent Transportation Systems (ITSC), Rhodes, Greece, 2020, pp. 1-5, doi: 10.1109/ITSC45102.2020.9294505.
- P. Held, D. Steinhauser, A. Kamann, A. Koch, T. Brandmeier, and U. T. Schwarz, “Normalization of Micro-Doppler Spectra for Cyclists Using High-Resolution Projection Technique,” in 2019 IEEE International Conference on Vehicular Electronics and Safety (ICVES), Cairo, Egypt, 2019, pp. 1-6, doi: 10.1109/ICVES.2019.8906495.
- K. Schneider, R. Lugner, and T. Brandmeier, “LiDAR-Based Contour Estimation of Oncoming Vehicles in Pre-Crash Scenarios,” in 2019 IEEE Intelligent Vehicles Symposium (IV), Paris, France, 2019, pp. 2272-2277, doi: 10.1109/IVS.2019.8813825.
- P. Zaumseil, D. Steinhauser, P. Held, A. Kamann, and T. Brandmeier, “Radar-based Near Field Environment Perception using Back Projection Algorithm,” in 2019 16th European Radar Conference (EuRAD), Paris, France, 2019, pp. 257-260, Paris, France.
- G. J. Sequeira, S. Afraj, R. Lugner, and T. Brandmeier, “LiDAR Based Prediction and Contact Based Validation of Crash Parameters for a Preemptive Restraint Strategy,” in IEEE International Conference of Vehicular Electronics and Safety (ICVES), Cairo, Egypt, 2019, pp. 1-7, doi: 10.1109/ICVES.2019.8906354.
- P. Held, D. Steinhauser, A. Kamann, A. Koch, T. Brandmeier, and U. T. Schwarz, "Micro-Doppler Extraction of Bicycle Pedaling Movements Using Automotive Radar," in 2019 IEEE Intelligent Vehicles Symposium (IV), Paris, France, 2019, pp. 744-749, doi: 10.1109/IVS.2019.8813828.
- G. J. Sequeira, K. Schneider, R. Lugner, and T. Brandmeier, “Concepts for Validation Sensor for Pre-Crash Information from Forward-Looking Sensors,” in Sensors and Measuring Systems; 19th ITG/GMA-Symposium, Nuremburg, Germany, 2018, pp. 1-5.
- A. Kamann, P. Held, F. Perras, P. Zaumseil, T. Brandmeier, and U. T. Schwarz, “Automotive Radar Multipath Propagation in Uncertain Environments,” in 2018 21st International Conference on Intelligent Transportation Systems (ITSC), Maui, HI, USA, 2018, pp. 859-864, doi: 10.1109/ITSC.2018.8570016.
Contact
Prof. Dr.-Ing. Thomas Brandmeier
Phone: +49 841 9348-3840
Room: H023
E-Mail: Thomas.Brandmeier@thi.de
Dr. rer. nat. Dagmar Steinhauser
Phone: +49 841 9348-3375
Room: H120
Fax: +49 841 9348-993375
E-Mail: Dagmar.Steinhauser@carissma.eu