Summary:
Learning algorithms offer in battery systems new possibilities for innovative battery management. Due to the complex non-linear system behavior and the large number of sensor data (voltage, current, temperature, pressure, ...), the methods of artificial intelligence unfold their full potential, especially in this application area. On the basis of physically motivated white-box models and data-driven black-box models, the simulative mapping of the real system behavior takes place. Recursive estimation methods such as the Kalman filter or the recursive least squares algorithm provide a precise state estimation in the cell network. By using additional state observers or neural networks in the adaptive models, a fault diagnosis in the battery system can be implemented, as well. The research is not limited to real-time-capable online methods, e.g. directly in the vehicle, but also includes cloud-based approaches in the back-end.
In addition, the research group ELS investigates methods of machine learning for the safe operation of large battery systems. Therefore, a key issue is the prediction of the lifetime and the detection of safety-relevant events. In this case, for example, the use of swarm intelligence of connected electric vehicles and self-learning algorithms provide realistic prediction models. Based on this, optimal operation of the battery system is achievable.
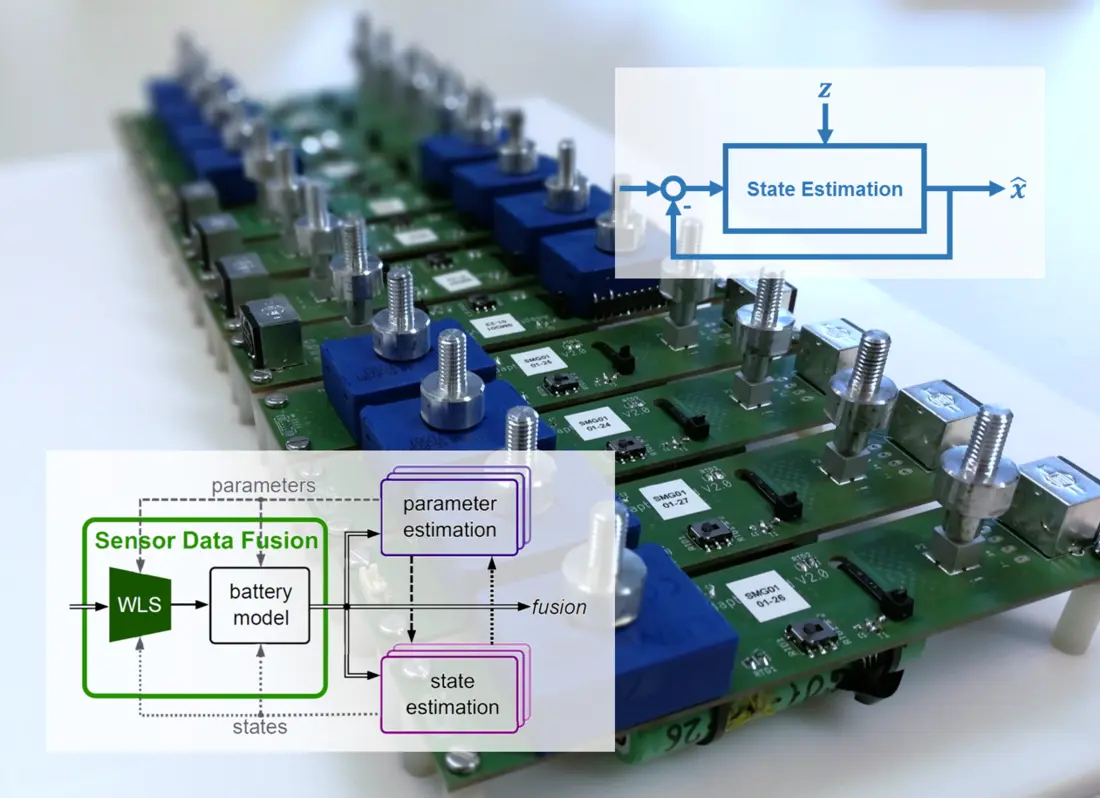
Contact
Christoph Terbrack, M.Sc.
Phone: +49 841 9348-6487
Room: S421
Fax: +49 841 9348-996487
E-Mail: Christoph.Terbrack@thi.de